Materials and devices as solutions to computational problems in machine learning
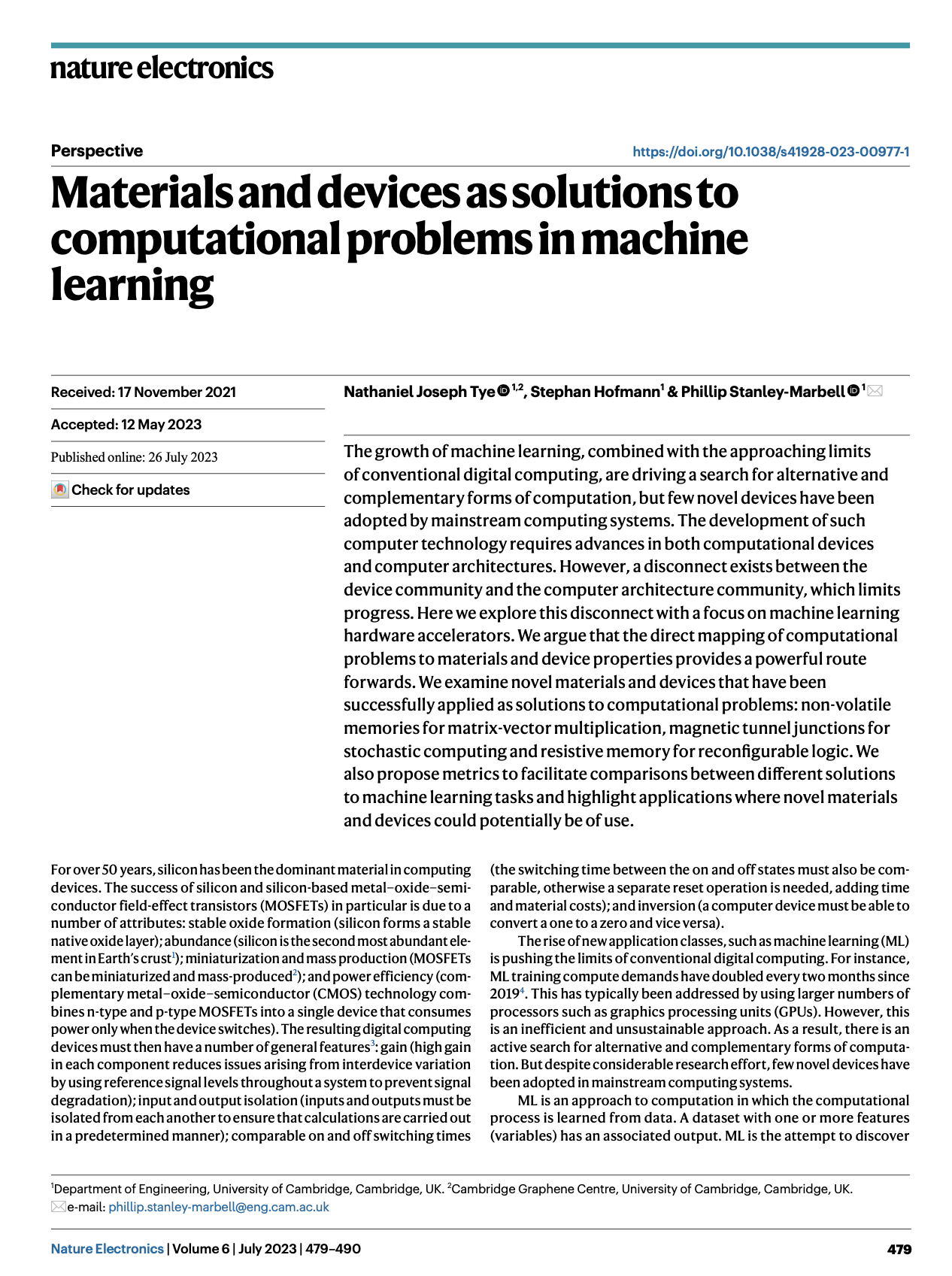
Nathaniel Joseph Tye, Stephan Hoffman and Phillip Stanley-Marbell, Nature Electronics, 26 July, 2023.
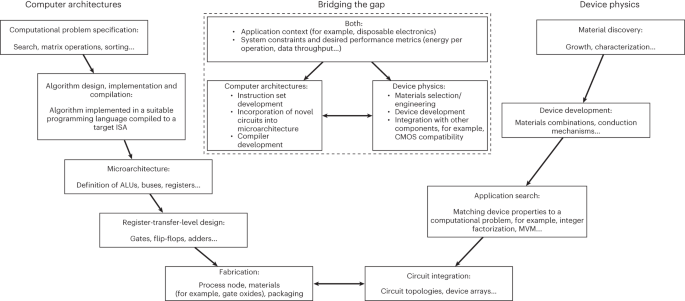
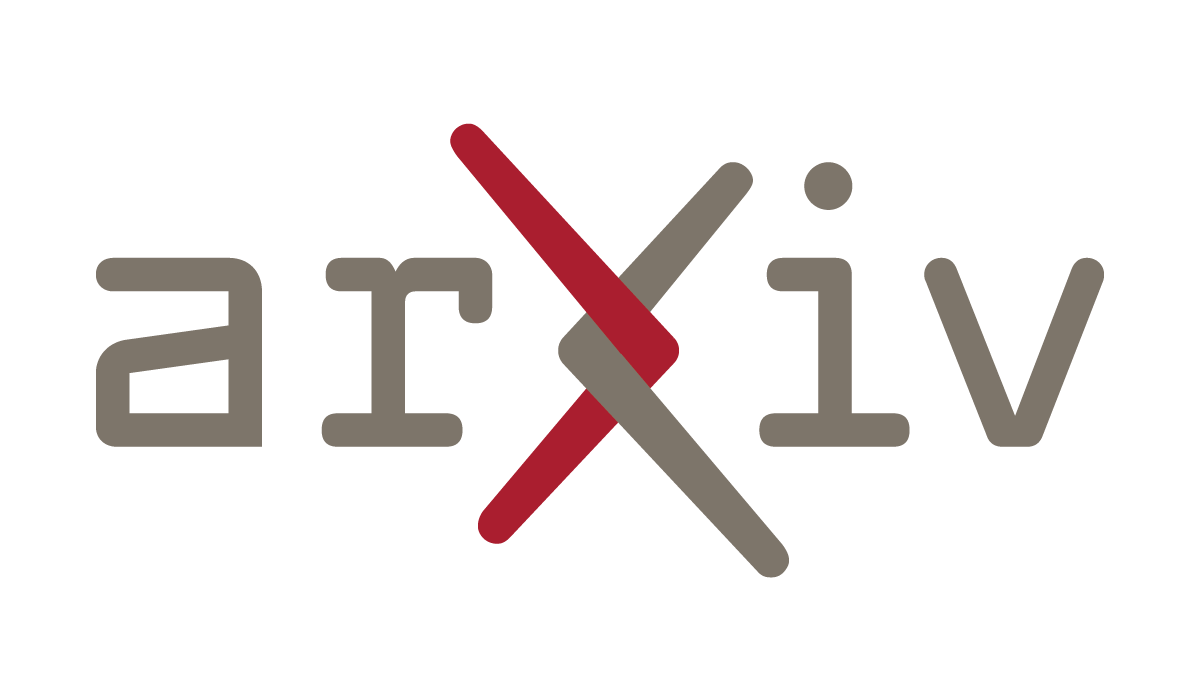
Abstract
The growth of machine learning, combined with the approaching limits of conventional digital computing, are driving a search for alternative and complementary forms of computation, but few novel devices have been adopted by mainstream computing systems. The development of such computer technology requires advances in both computational devices and computer architectures. However, a disconnect exists between the device community and the computer architecture community, which limits progress. Here we explore this disconnect with a focus on machine learning hardware accelerators. We argue that the direct mapping of computational problems to materials and device properties provides a powerful route forwards. We examine novel materials and devices that have been successfully applied as solutions to computational problems: non-volatile memories for matrix-vector multiplication, magnetic tunnel junctions for stochastic computing and resistive memory for reconfigurable logic. We also propose metrics to facilitate comparisons between different solutions to machine learning tasks and highlight applications where novel materials and devices could potentially be of use.
Cite as:
Tye, N.J., Hofmann, S. & Stanley-Marbell, P. Materials and devices as solutions to computational problems in machine learning. Nat Electron6, 479–490 (2023). https://doi.org/10.1038/s41928-023-00977-1
Bibtex:
@article{tye2023materials,
title={Materials and devices as solutions to computational problems in machine learning},
author={Tye, Nathaniel Joseph and Hofmann, Stephan and Stanley-Marbell, Phillip},
journal={Nature Electronics},
volume={6},
number={7},
pages={479--490},
year={2023},
publisher={Nature Publishing Group UK London}
}